
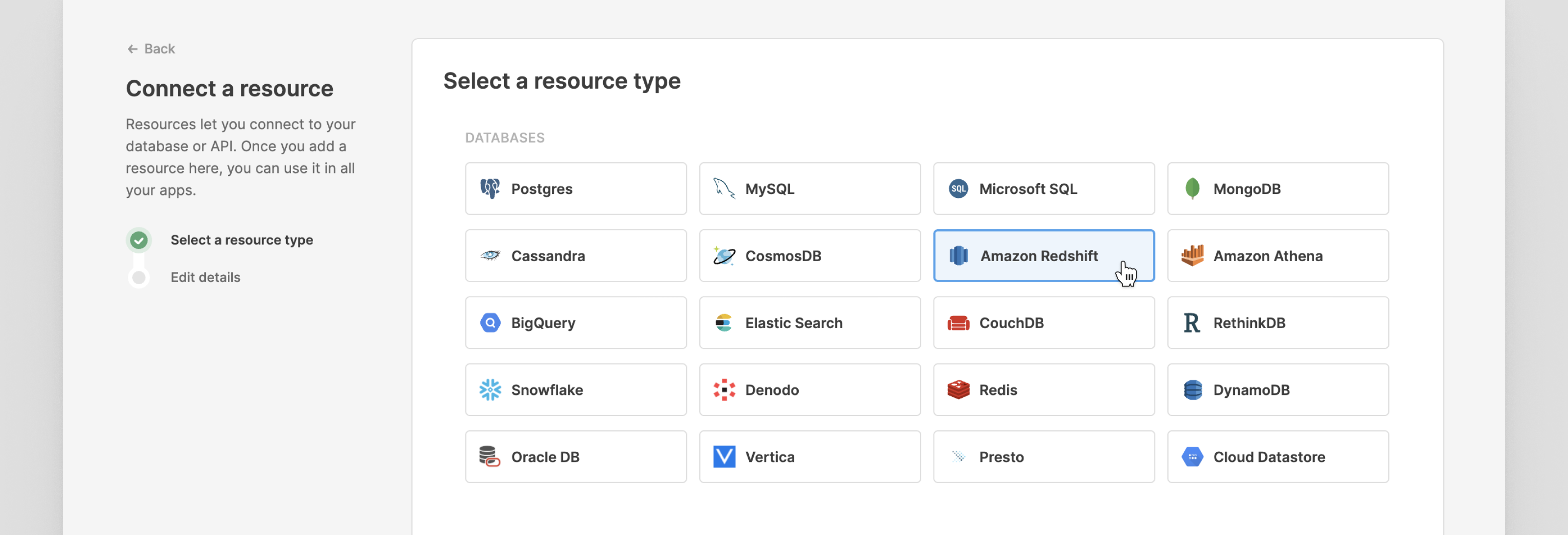
If a full table scan is required and each record needs to be calculated for the date function it can make queries extremely expensive.Īlthough Redshift is advertised as having almost the same features as PostgreSQL there is one big missing feature that make it very difficult to generate a date dimension table: Once you start performing date calculations it’s very unlikely the database will be able to make optimisations or smart decisions that allow it to use an index.Date calculations are complicated in the easiest cases and making sure you handle all the edge cases is very complicated and error prone.Trying to do these date calculations in your queries raises a few problems: All days except the last day of each month.How would you go about handling reports that needed to filter or summarise (group) on? Let’s say we have a table that contains sales information lot’s of it. Used in combination the date dimension table these two integer indexes represent an exact second for any date.Įach will have their own separate table that contains the date/time ID and any information about that particular day or second. Usually picking a date at or slightly before the earliest records in your database is a good choice.Ī time dimension table is similar in that it will assign an index to individual seconds for one day (but have no date component). Where that starting point is depends on how far back you will need to go.
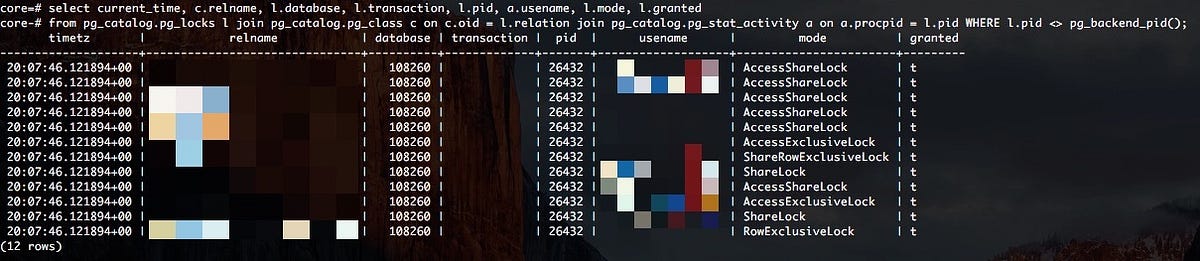
It is common practice in data warehousing and reporting to use date and time dimension tables.Ī date dimension table assigns an index to each day from an arbitrary starting point. The Identify method ties user attributes to a userId.Photo by Estée Janssens on Unsplash What Is It? tracks ) last ) final Identify users Historical traits tracks ) last WHERE EXTRACT ( 'EPOCH' FROM sent_at ) - EXTRACT ( 'EPOCH' FROM last_event ) >= ( 60 * 10 ) OR last_event IS NULL - Mapping every event to its session SELECT *, SUM ( is_new_session ) OVER ( ORDER BY user_id, sent_at ROWS BETWEEN UNBOUNDED PRECEDING AND CURRENT ROW ) AS global_session_id, SUM ( is_new_session ) OVER ( PARTITION BY user_id ORDER BY sent_at ROWS BETWEEN UNBOUNDED PRECEDING AND CURRENT ROW ) AS user_session_id FROM ( SELECT *, CASE WHEN EXTRACT ( 'EPOCH' FROM sent_at ) - EXTRACT ( 'EPOCH' FROM last_event ) >= ( 60 * 10 ) OR last_event IS NULL THEN 1 ELSE 0 END AS is_new_session FROM ( SELECT *, LAG ( sent_at, 1 ) OVER ( PARTITION BY user_id ORDER BY sent_at ) AS last_event FROM "your_source". Finding the start of every session SELECT * FROM ( SELECT * LAG ( sent_at, 1 ) OVER ( PARTITION BY user_id ORDER BY sent_at ) AS last_event FROM "your_source". Mode walks you through the reasoning behind the query, what each portion accomplishes, how you can tweak it to suit your needs, and the kinds of further analysis you can add on top of it. Here’s the query to make it happen, but read Mode Analytics’ blog post for more information. To define sessions with raw SQL, a great query and explanation comes from Mode Analytics. Segment recommends checking out Looker’s approach here. With Looker, for example, you can take advantage of their persistent derived tables and LookML modeling language to layer sessionization on top of your Segment SQL data.
Redshift current date how to#
How to define user sessions using SQLĮach of Segment’s SQL partners allow you to define sessions based on your specific business needs. Segment leaves session interpretation to SQL partners, which let you design how you measure sessions based on how customers use your product.įor more on why Segment doesn’t collect session data at the API level, check out a blog post here. And since Segment’s API is about collecting raw, factual data, there’s no API for collecting sessions. They’re stories Segment builds around the data to understand how customers actually use the product in their day-to-day lives. Sessions aren’t fundamental facts about the user experience. Segment’s API does not impose any restrictions on your data with regard to user sessions. That query returns a table like this: date
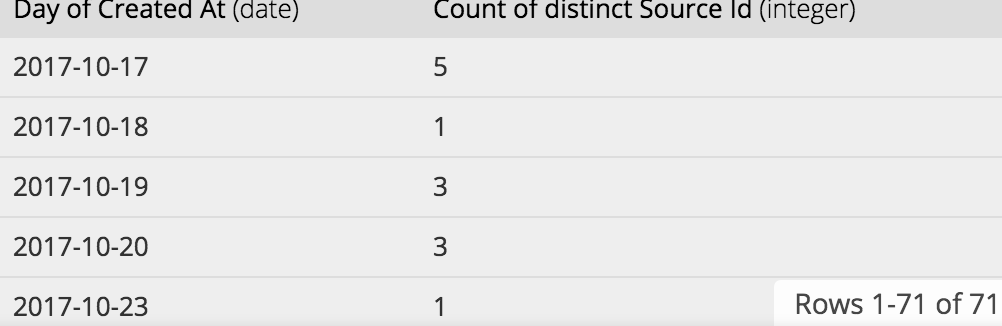
tracks where event = 'completed_order' group by date
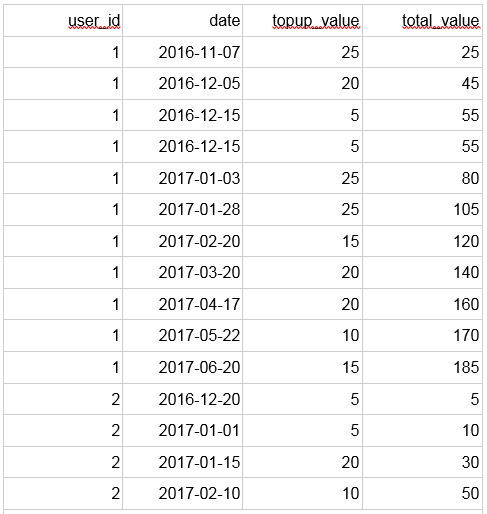
Select date ( sent_at ) as date, sum ( case when item = 'shirt' then 1 else 0 end ) as shirts, sum ( case when item = 'pants' then 1 else 0 end ) as pants from initech.
