
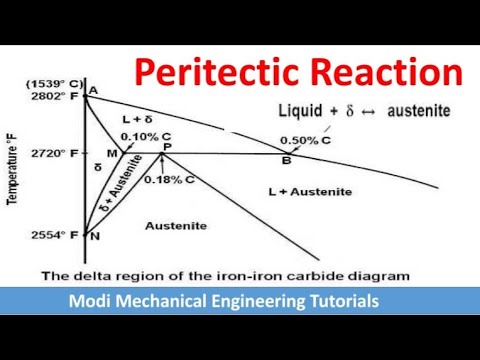
Wang L, Apelian D, Makhlouf M, Huang W (2008) Predicting compositions and properties of aluminum die casting alloys using artificial neural network.Int Symp Form Sci Eng 2:428-432 Google Scholar Digital Library Shang GQ (2008) Application of BP neural network for predicting anode accuracy in ECM.Adv Neural Netw, Lecture Notes in Computer Science 3174:262-268 Google Scholar Cross Ref Pang G, Xu W, Zhai X, Zhou J (2004) Forecast and control of anode shape in electrochemical machining using neural network.Electrochim Acta 53(4):1877-1882 Google Scholar Cross Ref Parthiban T, Ravi R, Kalaiselvi N (2007) Exploration of artificial neural network to predict the electrochemical characteristics of lithium-ion cells.Bhattacharyay D, Kocaefe D, Kocaefe Y, Morrais B (2015) Comparison of linear multivariable, partial least square regression, and artificial neural network analyses to study the effect of different parameters on anode properties.Bhattacharyay D, Kocaefe D, Kocaefe Y, Morrais B, Gagnon M (2013) Application of the artificial neural network (ANN) in predicting anode properties.Boadu KD, Omani FK (2010) Adaptive control of feed in the Hall-Héroult cell using a neural network.2nd international conference on information science and engineering (ICISE), pp 1456-1459 Google Scholar Li J, Zhou H, Guo T (2010) Research on fault diagnosis method based on modified elman neural network.Metall Mater Trans B 29(5):1007-1019 Google Scholar Cross Ref Meghlaoui A, Bui RT, Thibault J, Tikasz L, Santerre R (1998) Predictive control of aluminum electrolytic cells using neural networks.Biedler P, Banta L, Dai C, Love R, Tommey C, Berkow J (2002) Development of a state observer for an aluminum reduction cell.Int J Hydrogen Energy 34(13):5546-5553 Google Scholar Cross Ref Milewski J, ¿wirski K (2009) Modelling the SOFC behaviours by artificial neural network.Dissertation, Norwegian University of Science and Technology Google Scholar Chmelar J (2006) Size reduction and specification of granular petrol coke with respect to chemical and physical properties.Berezin AI, Polaykov PV, Rodnov OO, Klykov VA, Krylov VL (2002) Improvement of green anodes quality on the basis of the neural network model of the carbon plant workshop.The predictions were found to be in good agreement with the results of other studies in the literature. The effect of CO2 and air reactivities of coke on the CO2 reactivity of anode was also studied. ANN also showed the effect of pitch percentage and coke porosity on the CO2 reactivity of anodes. The artificial neural network was also used to identify the effect of impurities such as, vanadium, iron, sodium, and sulfur on the CO2 reactivity of anodes.

The average error between the predicted and experimental values is around 6 %. In this study, published data have been used to show the proficiency of different artificial neural networks using the MATLAB software. In such situations, the artificial neural network (ANN) methods can serve as a useful tool to predict anode properties. Usually, no well-known mathematical relationship exists between the various physical and chemical properties of raw materials and the final anode properties. The impurities in the raw materials, which are mainly by-products of different industries, influence significantly the quality of anodes. It is mainly composed of calcined petroleum coke, coal tar pitch, and recycled carbon materials. Carbon anode is one of the key components for the electrolytic production of aluminum.
